Organizations have seen an exponential increase in end user and IoT devices driven by productivity. Critical to the performance of these devices is their ability to access a plethora of SaaS applications, including real-time voice and video and several network services.
The modern network of today is delivered as a cloud-first service over a wireless-first infrastructure. Device heterogeneity and device mobility, combined with a high transaction and data volume, have made the network far more dynamic and the operations more complex at the edge. Legacy management solutions were designed to manage static networks used by a limited set of IT sanctioned clients and applications. These outdated solutions lack coherent and meaningful insights from a client context. If a client has issues with application performance, it is hard to pinpoint if the problem is systemic or isolated, a result of poor Wi-Fi performance, an impairment on WAN, a problem with network services, or an issue with the application. IT teams cannot rely on these antiquated tools to ensure that the modern day clients get the best performance from the network infrastructure, network and security services, and applications. IT teams, and the organizations they support, require advanced analytics and actionable intelligence made possible by an AIOps solution.
VMware Edge Intelligence: A Comprehensive AIOps Solution
VMware Edge Intelligence is a vendor agnostic AIOps solution focused on the enterprise edge that ensures the end user and IoT client performance, security, and self-healing through wireless and wired LAN, SD-WAN, and secure access service edge (SASE).
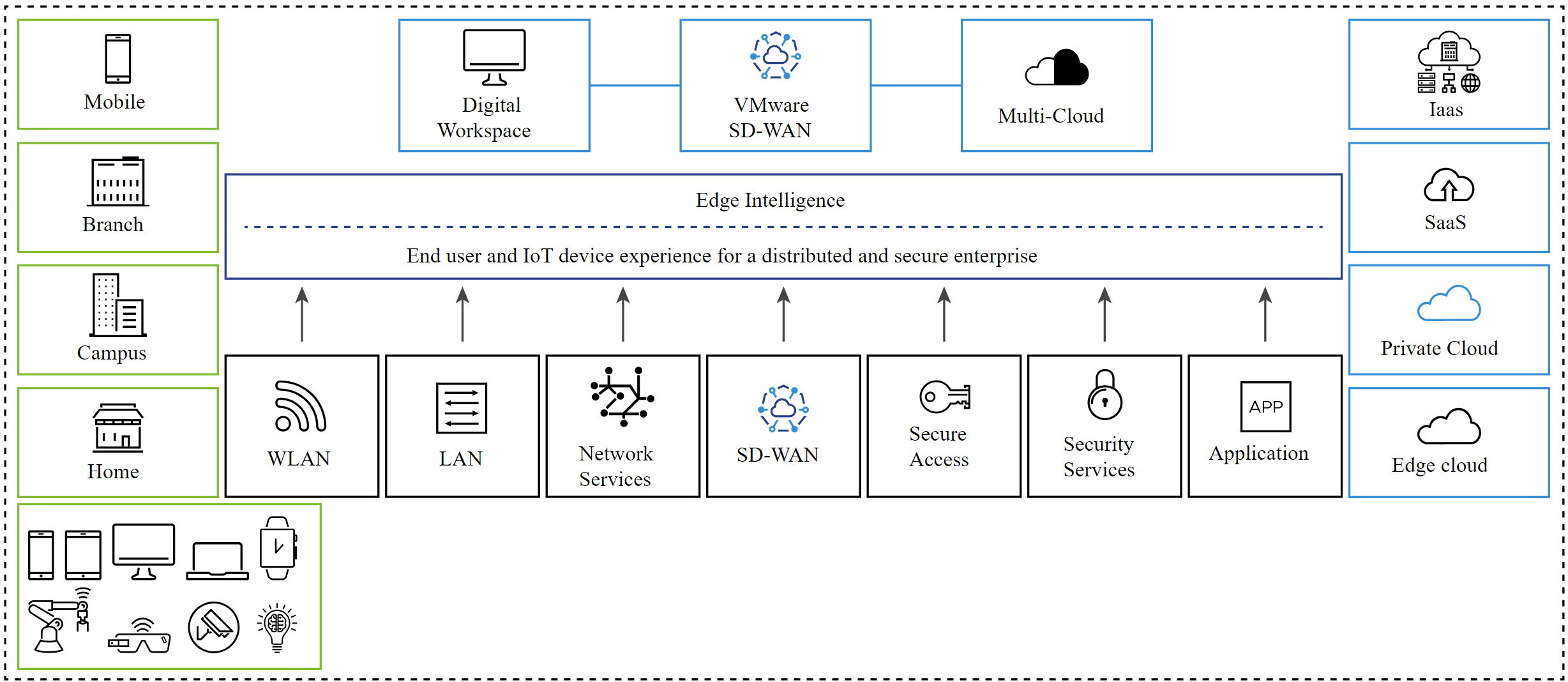
Key Highlights
-
An AIOps solution focused on the enterprise edge. Ensures end user and IoT client performance, security, and self-healing through wireless and wired LAN, SD-WAN and SASE.
-
Customers have seen from 40 to 55% improvement in the client device productivity by deploying this solution.
-
Accelerates transformation of IT Operations to a proactive mode.
Solution Benefits
-
Rich Client Experience
VMware Edge Intelligence significantly improves and quantifies the end user & IoT device experience at any location. With a distributed workforce, IT teams lack visibility into the client experience at every location. They need a solution that reduces the need for IT visits. VMware Edge Intelligence can pinpoint with clarity whether a perceived application problem is due to issues with the local Wi-Fi network, broadband network, WAN, network services or with the application. Customers using VMware Edge Intelligence have seen 40 to 55% improvement in client device productivity.
-
Proactive Remediation
VMware Edge Intelligence uses ML techniques, including nearest neighbor and unsupervised clustering algorithms, to automatically isolate faults, identify root cause, make recommendations, and predict benefits. Using internal and industry benchmarks, the solution provides opportunities for continuous improvements. Using VMware Edge Intelligence, customers have seen a 50% reduction in time to find and fix the issues.
-
Application Assurance
Enterprises use hundreds of SaaS applications. While a small percentage of these applications is sanctioned by IT, most productivity applications are not. Tracking these applications becomes untenable for IT teams. VMware Edge Intelligence provides assurance for over 3000 applications with contextual performance indicators like the number of clients affected or number of sites impacted.
-
Operational Simplicity
VMware Edge Intelligence currently detects and analyzes over 30 million devices. The wide variety and growth in the number of devices makes it impossible to manually identify, classify, and analyze the performance. The solution uses time-series based ML algorithms to auto-discover these devices and establish a baseline for monitoring.